Disadvantages of AI in Business: Risks You Should Know
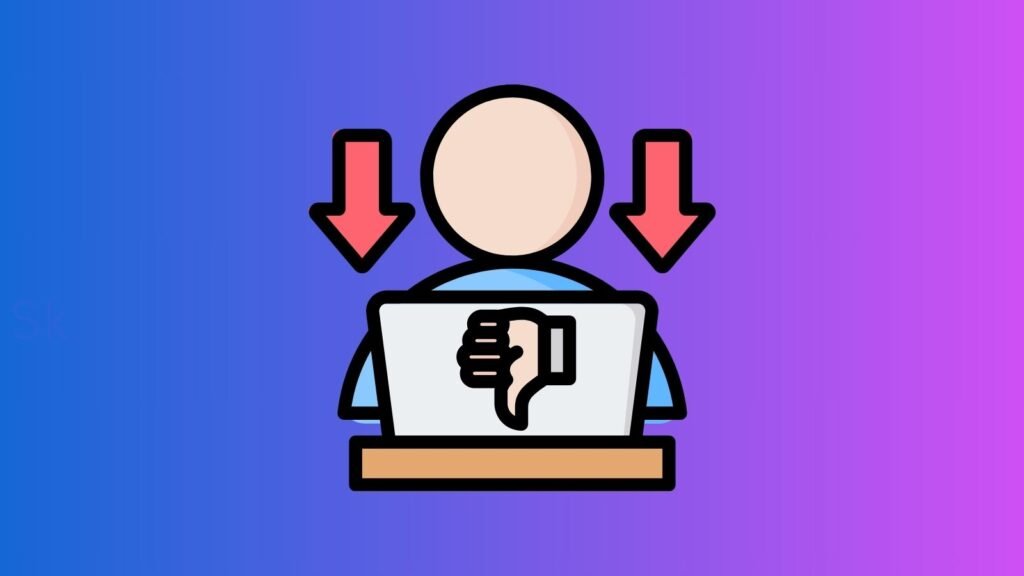
Are you thinking about using artificial intelligence (AI) in your business? AI can be very helpful, but there are also big disadvantages of AI in business that you need to know about.
This post will explore the main risks and downsides of using AI systems. It will help you make smart decisions about using this powerful technology properly in your organization.
We’ll cover issues like data privacy concerns, risks of people losing jobs, and ethical challenges with AI. You’ll learn valuable information to understand and deal with the AI terrain. Keep reading to learn about the key disadvantages of AI in business that every leader should know.
Key Takeaway – Disadvantages of AI in Business
- AI poses risks like data privacy breaches, job displacement, and ethical challenges.
- Bias in AI algorithms and lack of transparency can lead to unfair decisions.
- High implementation costs and integration challenges complicate AI adoption.
- Human oversight and responsible practices are crucial to mitigate AI risks and ensure ethical use.
Data Privacy and Security Concerns
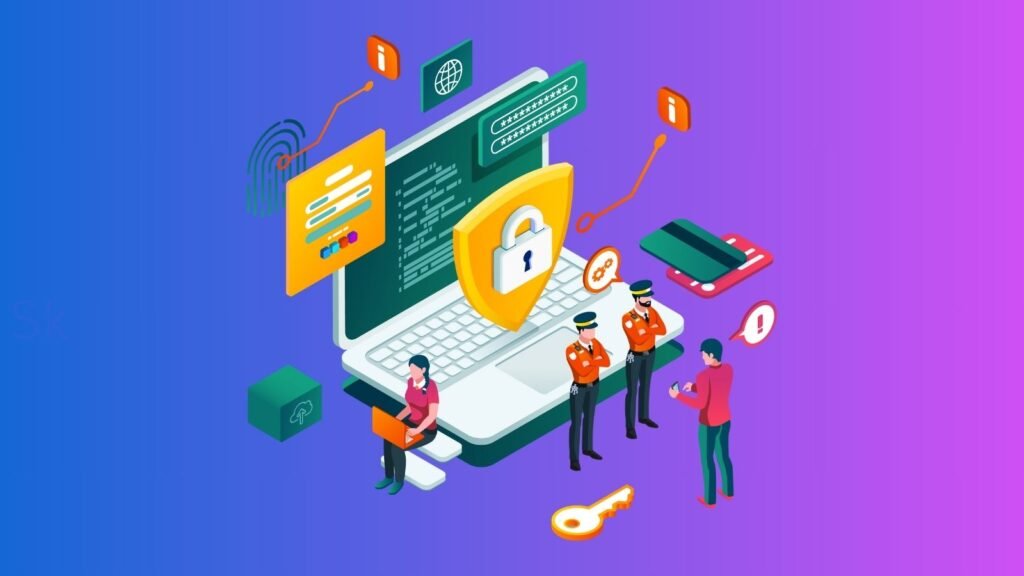
Handling Sensitive Customer Data
Companies using artificial intelligence must be careful with customer data, especially private info like bank records or patient data. AI algorithms need huge amounts of data to learn from (training data).
This data could accidentally expose private customer info if not properly protected. Strict data rules and strong cybersecurity are needed to prevent data breaches and keep customers’ trust.
Potential for Data Breaches & Misuse
The way AI systems centralize data creates risks of data misuse if the systems get hacked. Bad actors could get unauthorized access to private training data, and AI models, or even control the AI itself for harmful purposes.
Businesses must invest in serious security protocols and ongoing maintenance to reduce these threats and protect their valuable insights from AI.
Ethical Considerations in Data Collection & Usage
Beyond security risks, there are ethical issues with how data is collected and used for AI development. Concerns over data privacy, consent, and possible bias in datasets could damage public trust in adopting AI.
Businesses should prioritize transparency, establish clear ethical guidelines, and involve diverse groups to responsibly navigate these complex topics.
Job Displacement and Workforce Implications
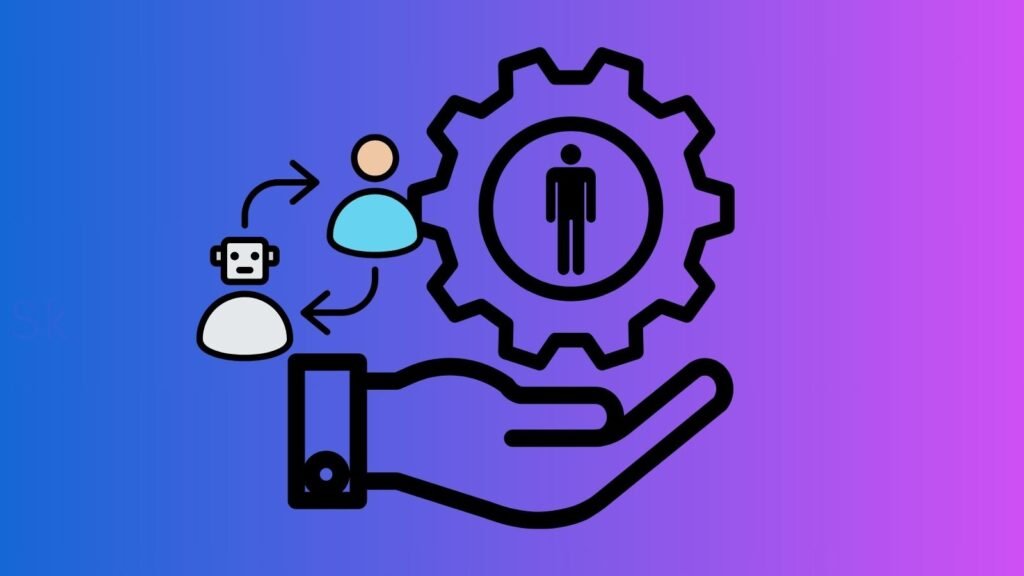
AI’s Potential to Replace Human Workers
One of the biggest concerns around adopting AI is the risk of job displacement. As businesses increasingly automate repetitive tasks with AI systems, human workers across various industries could lose their jobs to AI automation.
While AI may enhance efficiency, the potential job losses raise concerns about the long-term societal impact of general AI adoption.
Impact on Specific Industries & Job Roles
Certain sectors are more susceptible to AI automation than others. Manufacturing, transportation (including self-driving cars), customer service (virtual assistants, AI-powered chatbots), and data entry roles that involve repetitive tasks are at higher risk of being disrupted by AI capabilities that can perform tasks more efficiently.
Even highly skilled professions like accounting, radiology, and software development may need help as AI gets better at analyzing data and identifying patterns.
Challenges in Retraining & Reskilling Workforce
As AI increasingly takes over certain routine tasks, there will be an urgent need to retrain and reskill impacted workers.
However, this process poses significant challenges in terms of cost, time, and developing comprehensive training programs to transition employees to new roles that require human creativity and skills that AI cannot easily replicate.
Businesses and governments must proactively address these workforce implications to avoid general job displacement.
Bias and Ethical Issues
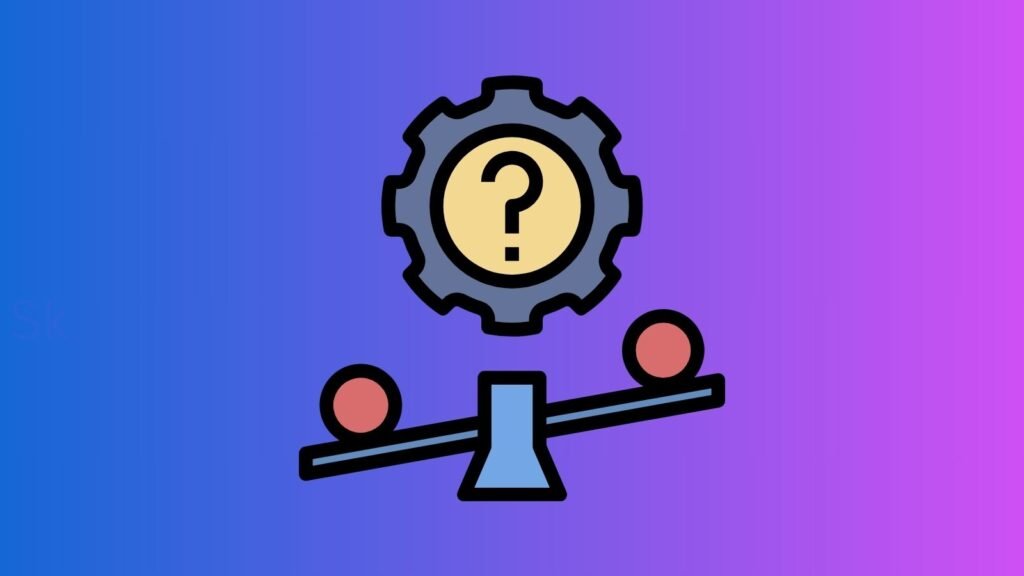
Bias in Training Data & AI Algorithms
One major disadvantage of artificial intelligence (AI) is the possibility of bias. This bias can come from the data used to teach the AI algorithms (training data). If the data is biased or lacks diversity, the AI models created from it may make biased decisions when analyzing data or making decisions.
Biased AI could lead to unfair treatment, especially in important areas like hiring, lending, and law. Carefully checking training data and algorithms for bias is very important.
Lack of Transparency & Accountability
Many AI technologies, especially advanced machine learning algorithms, are like “black boxes” – their inner workings are hidden, even to their creators.
This lack of transparency raises accountability issues – it’s hard to explain AI outputs or say who is responsible when things go wrong. As businesses lean more on AI for critical tasks, ensuring there’s transparency and accountability in how AI operates will be essential.
Ethical Concerns in AI Decision-Making
As AI capabilities advance, the decisions and judgments made by AI systems will have far-reaching real-world effects influencing human lives. However, current AI lacks the strong ethical reasoning abilities that humans employ.
How do we ensure AI adheres to moral and ethical principles when making decisions that could harm individuals’ rights, dignity, or well-being? Businesses face the challenge of creating ethical AI, but many are finding real-world success in its implementation. (How Businesses Are Using AI: Real-World Success Stories)
Dependence on High-Quality Data
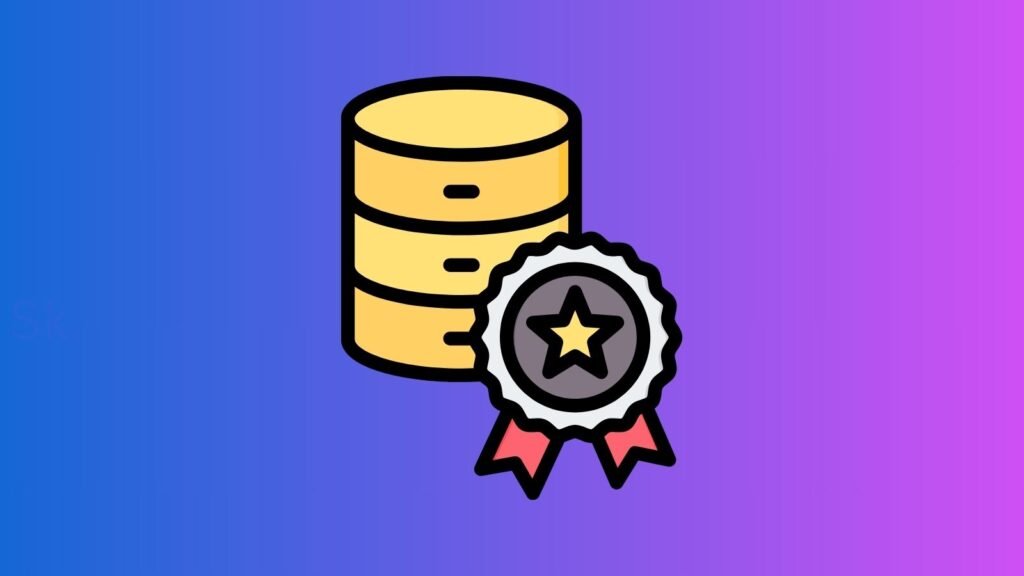
Importance of Accurate & Unbiased Data
The significance of AI systems mainly depends on the quality of the data used to prepare them. Wrong, incomplete, or biased training data can lead to flawed models that make incorrect informed decisions or produce obligatory consequences.
Businesses must ensure they have access to high-quality data that is expected, up-to-date, and free from inherent biases to fully leverage AI’s power.
Challenges in Data Collection & Preprocessing
Getting clean, reliable data for training data is often very difficult. Data collection can be hard work, expensive, and lead to issues like unstable formats, missing information, and duplicates.
Even after collection, a lot of data preprocessing is needed to structure, clean, and properly label the data before it can be used for AI development. These upfront data preparation challenges make successfully executing AI harder.
Consequences of Using Low-Quality or Incomplete Data
If businesses utilize AI models built on low-quality training data, the resulting AI-powered tools will likely underperform or make mistaken decisions that could disrupt operations.
Incomplete data may cause blind spots, causing AI to miss important factors when analyzing data. Simply put, low-quality data means low-quality AI outputs, threatening any hoped-for benefits like cost savings, competitive edge, or better processes.
Implementation and Integration Challenges
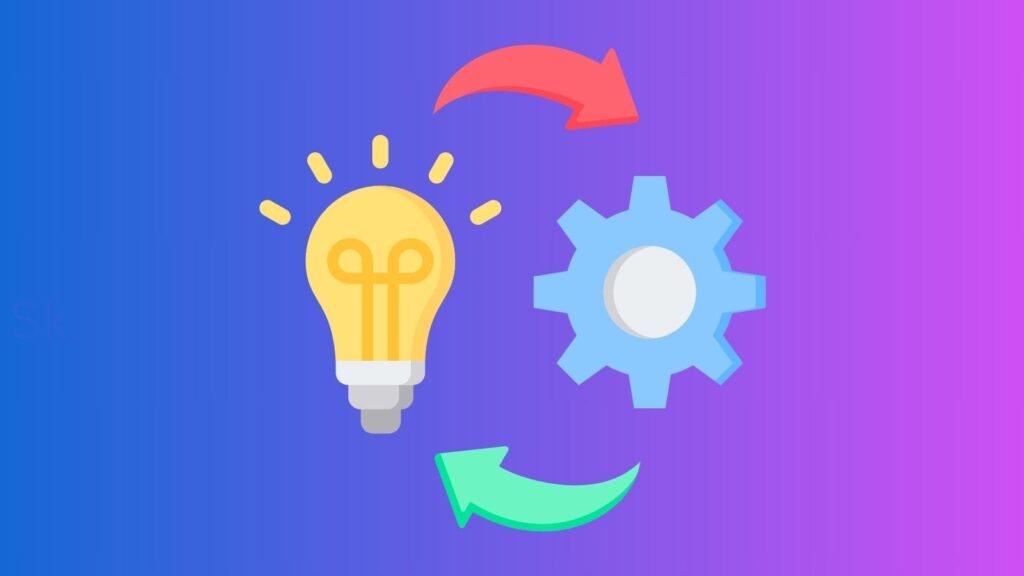
Initial Implementation Costs & Resource Requirements
If businesses utilize AI models built on low-quality training data, the resulting AI-powered tools will likely underperform or make mistaken decisions that could disrupt operations.
Incomplete data may cause blind spots, causing AI to miss important factors when analyzing data. While poor data can hinder AI performance, businesses can discover numerous benefits when implemented correctly. (“What Can AI Do for My Business? Discover the Benefits of AI”)
Integration with Existing Systems & Processes
For businesses that already have older computer systems and processes in place, combining new AI technologies is not easy. The AI tools may not work well with the older software, databases, and business processes.
Getting AI to work requires extensive customization, modifying existing code, moving data, and re-engineering how things operate. These complex integration challenges make AI rollouts longer, harder, and more expensive within an organization.
Ongoing Maintenance & Updates
Implementing AI is not a one-time thing – it requires continuous effort. As AI models age, they become less accurate due to changes and shifts in data.
Companies need to invest in always monitoring and retraining AI algorithms with new data, ensuring their systems remain correct and up-to-date over time.
Regulation changes may also require revising AI policies periodically. Managing this ongoing AI maintenance creates additional costs and staffing needs.
Limitation of AI Capabilities
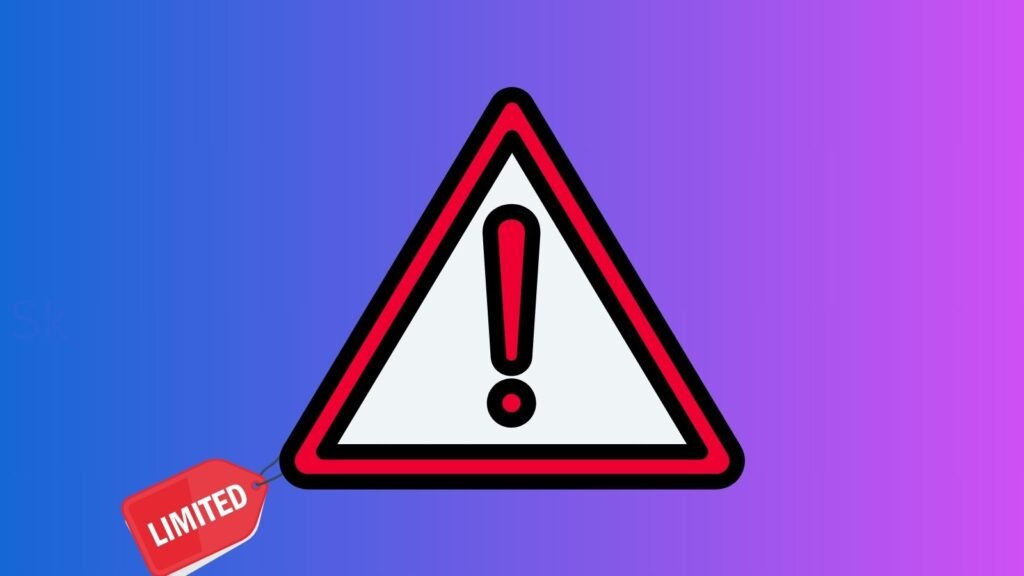
Tasks Requiring Human Creativity & Emotional Intelligence
While AI excels is great at automating repetitive tasks, analyzing vast amounts of data, and identifying patterns, it currently can’t match human skills in creative thinking, emotional intelligence, and general reasoning.
Creative work like art, writing, strategic planning, and marketing often require human creativity that AI can’t fully replicate.
AI works with nuanced communication, empathy, and emotional support, which are important in roles like counseling, social work, and customer service—areas where human workers truly shine.
Inability to Handle Unpredictable or Ambiguous Situations
Current AI technologies are very good at following specific rules and instructions. However, they have difficulty with unclear, unpredictable situations that require flexible problem-solving and contextual understanding.
For example, self-driving cars may have trouble with unexpected road hazards or detours they haven’t been trained for. AI assistants like virtual assistants and chatbots can get confused by open-ended questions that aren’t in their scripts.
Human intelligence is still essential for handling complex and new situations.
Need for Human Oversight & Intervention
While AI can handle many tasks and offer valuable insights, it isn’t foolproof. AI systems can make errors, overlook important details, or generate flawed outcomes, particularly if they rely on biased or incomplete data.
Therefore, AI should be used as a supportive tool with human oversight, not as an all-knowing decision-maker. While human expertise remains important in certain fields, AI is showing in a new era of business and marketing. (“How Business and Marketing Are Changing with AI: A New Era”)
Regulatory and Legal Concerns
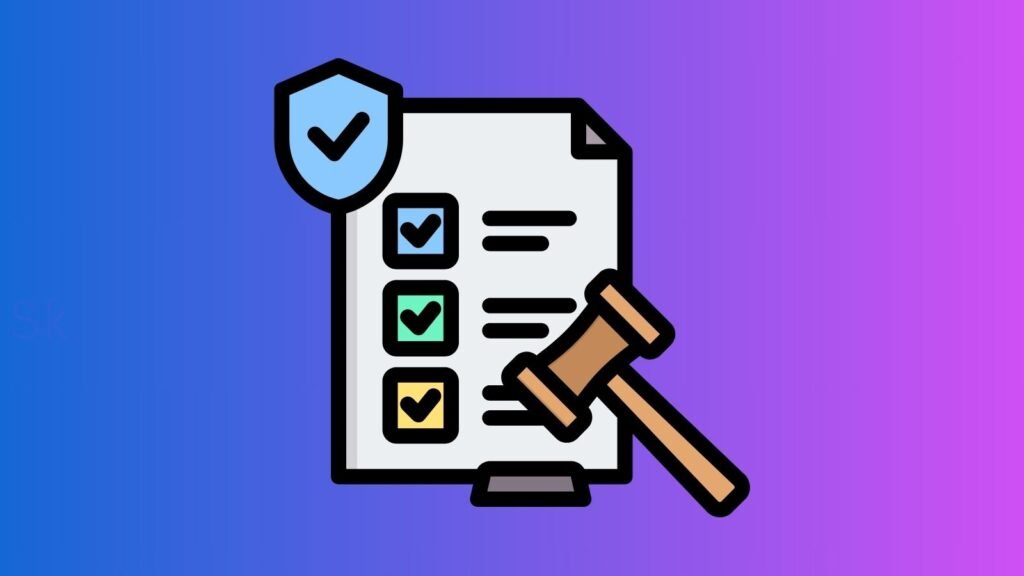
Data Privacy & AI Governance Regulations
As businesses leverage AI and manage large amounts of customer data, they must follow data privacy laws and new AI governance regulations. Strict regulations like GDPR and CCPA ensure people’s privacy by governing how their data is collected, used, and protected.
Governments are also creating new guidelines for ethical AI development, transparency in algorithms, and preventing bias in AI. Staying current with these constantly evolving regulations can be quite challenging.
Liability & Accountability Issues
When AI systems make mistakes or cause harm, figuring out who is responsible becomes very complicated because AI lacks clear accountability. If a self-driving car crashes, who is liable – the car maker, the AI developer, or the sensor manufacturer?
If biased AI leads to unfair lending decisions, is the financial institution or the third-party AI vendor responsible? As AI becomes more common, it will be important for businesses to clarify legal liability and insurance requirements around AI failures.
Intellectual Property Rights & Ownership
AI development often uses large training datasets, open-source libraries, and external AI models. This sharing of data and knowledge raises difficult questions about intellectual property (IP) ownership.
For example, if an AI model “learns” from copyrighted text, does it violate IP rights? Businesses using AI must carefully follow IP laws and licensing agreements and have clear data usage policies to avoid lawsuits or stifling innovation by keeping data too restricted.
Unintended Consequences and Risks
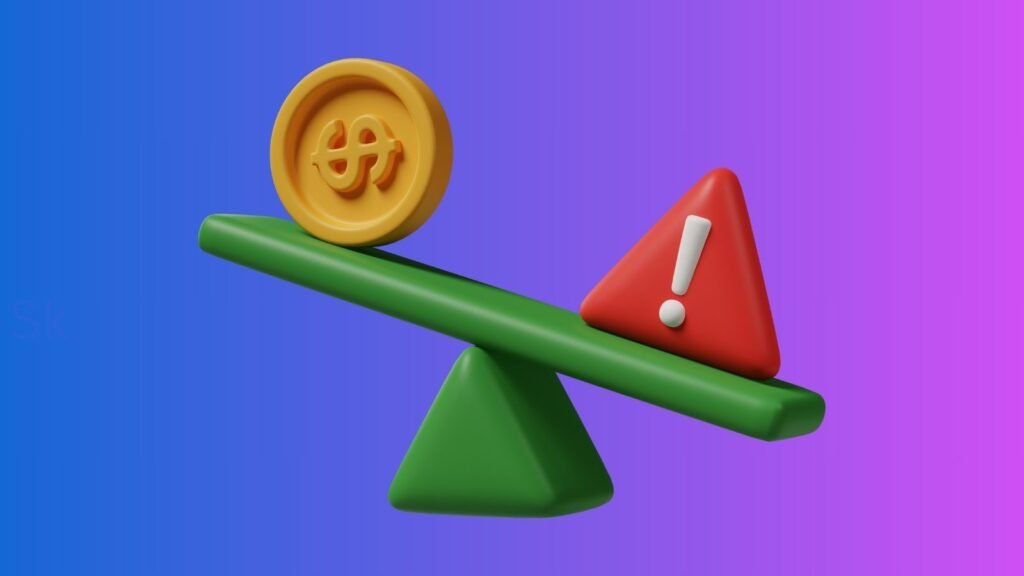
Potential for AI Systems to Make Mistakes or Cause Harm
Despite their increasing capabilities, AI systems are not perfect. They can make mistakes, miss important details, or produce flawed results, especially when trained on biased or incomplete data.
For instance, an inaccurate medical AI diagnostic tool could endanger patients, and malfunctioning self-driving car systems could cause accidents. Businesses must thoroughly test AI systems before using them to catch potential errors that could cause harm.
Lack of Human Control & Oversight
As AI becomes more independent and complex, there are worries about keeping adequate human control. Highly advanced AI might act in unexpected ways outside its intended purpose if not designed with strong safeguards.
Without proper human oversight and the ability to shut down runaway AI systems, they could cause problems through coding errors or malicious attacks. Ensuring AI systems are controllable is a critical challenge.
Ethical & Societal Implications of AI Adoption
The widespread adoption of AI raises important ethical questions we are just starting to understand. AI can perpetuate gender and racial biases and affect human identity through emotion-detecting technologies.
The societal impact of AI could be both positive and negative. There are also concerns about the existential risks if superintelligent AI systems do not align with human values and goals. It is crucial to guide these ethical implications together carefully.
Summary
While AI offers transformative possibilities for businesses, its general adoption comes with significant risks. These include data privacy breaches, job losses, bias in AI decisions, over-reliance on opaque systems, and misaligned ethical principles.
As AI capabilities grow, companies must proactively address these challenges through responsible practices, human oversight, and collaboration among various stakeholders. This approach ensures that AI benefits society without compromising core values.
Frequently Asked Questions
What Are the Main Risks of Using AI in Business?
Risks include data privacy issues, high costs, integration challenges, and potential biases in decision-making.
How Can AI Negatively Impact Jobs?
AI can automate tasks, potentially reducing the need for certain jobs and displacing workers.
Are There Privacy Concerns with AI in Business?
Yes, using AI often involves handling sensitive data, which can lead to privacy breaches if not properly managed.
How Expensive Is Implementing AI for Companies?
The cost can vary widely, but it often involves significant investment in technology, training, and maintenance.
Is AI Biased in Decision-Making?
AI can be biased if it learns from biased data, leading to unfair or discriminatory outcomes.
How Can Companies Mitigate AI Risks?
Companies can mitigate risks by ensuring data quality, implementing robust security measures, promoting transparency, and regularly auditing AI systems.